KIDSCORE PREDICTS IMPLANTATION AND CORRELATES WITH BLASTOCYST DEVELOPMENT AND QUALITY
The publication by Petersen et. al. "Development of a generally applicable morphokinetic algorithm capable of predicting the implantation potential of embryos transferred on Day 3" has been published in Human Reproduction. Read the entire publication here. On this page is an overview of the key messages and a short summary.
KIDScore predicts implantation with high accuracy using time-lapse
This algorithm was developed using a large multi-center dataset for predicting implantation with time-lapse assessment. Unlike some other published implantation prediction models, it is not clinic specific and therefore can be applied in any clinical setting with IVF and ICSI at high and low oxygen.
The algorithm* predicts the ability of an embryo to form a blastocyst much better than other published models and further predicts the clinically more important implantation rate with high accuracy.
As a deselection algorithm, KIDScore D3, has the advantage over selection algorithms that it does not risk ruling out embryos with a fair chance of implantation. This is important especially in cases where no top-scoring embryo is available – in those cases, KIDScore D3 makes possible the further consideration of less high-potential embryos.
*Algorithm described is the algorithm for KIDScore D3 which is part of the Vitrolife time-lapse portfolio
Summary
Objective
To develop a generally applicable and biologically meaningful deselection algorithm to predict implantation potential of embryos transferred on day 3 based on Known Implantation Data and data acquired through time-lapse monitoring of developing embryos.
Design
Retrospective analysis. Time-lapse data was collected from 3275 IVF/ICSI day 3 transferred embryos from cycles performed between 2009 and 2014 in 24 clinics. For each embryo the fate after transfer (implanted or not) was known.
Methods
The algorithm was developed as a decision tree and each step in the tree was decided based on the statistical method recursive partitioning considering a large set of candidate variables. The power of the developed algorithm was determined by ROC analysis and compared to other published algorithms by AUC-index.
Results
The resulting algorithm is designed in a way so that it does not make the decision for transfer, but rather ranks each embryo within a cohort according to their implantation potential. The final transfer/freeze choice is made by the embryologist who may include other criteria which are considered relevant.
The resulting algorithm identifies embryos with a very fast development into the 3-cell stage, embryos that are too slow to develop, embryos that show an irregular cleavage pattern (defined as two separate patterns with differing effect on embryo implantation potential), and embryos that do not reach a desirable stage of development on Day 3.
The AUC of the developed algorithm for implantation prediction is 0.650. When tested on an independent dataset with day 5 blastocyst data, the AUC for prediction of blastocyst development and of blastocyst quality, based on variables assessed until day 3, was 0.745 and 0.679, respectively. When tested for relative predictive power on multicenter day 5 data, out of the algorithms tested, KIDScore and one other algorithm performed best. Interestingly traditional embryo assessment was next in class after the two best time-lapse models (Figure 3).
Conclusions
The algorithm described has the potential to improve traditional embryo assessment and is a robust algorithm which includes only few parameters that are easy to assess in a consistent manner.
Key figures
Figure 1 of the publication shows the algorithm for KIDScore D3 which is designed as a decision tree.
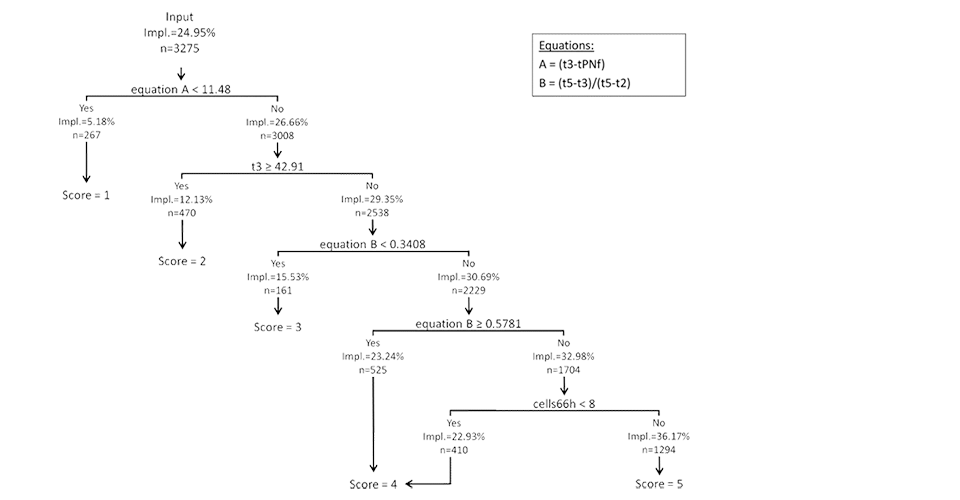
Figure 3 of the publication shows the relative predictive power of each of the algorithms compared in this study for blastocyst (A) and blastocyst quality (B). Only “KIDScore” and “Liu” show a higher degree of predictability than traditional morphology scoreing as represented by “Alpha/ESHRE”. Note that relative predictive power is a different meassure than absolute predictive power, which could only be calculated for KIDScore due to the inclusion of morphology in the other algorithms.
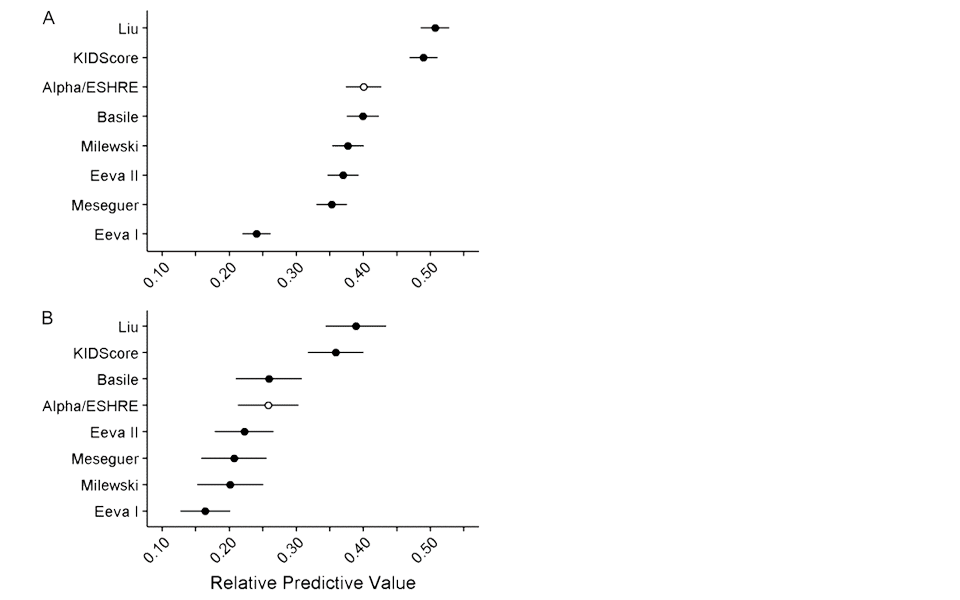